Introduction
The investment landscape has transformed dramatically with the advent of artificial intelligence (AI). Today, AI technologies enable investors to analyze vast datasets, predict market trends, and optimize their investment strategies more effectively than ever before. This comprehensive guide explores how to leverage AI to enhance your investment approach, equipping you with the tools and knowledge necessary to invest like an expert.
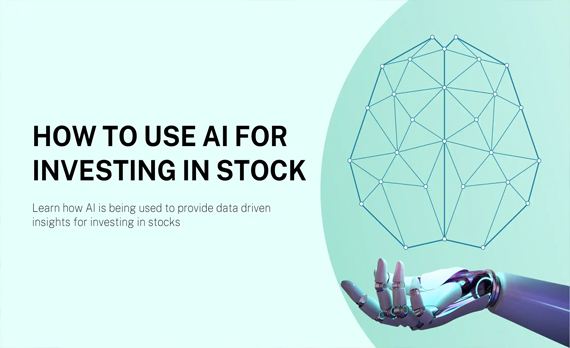
1. Understanding Artificial Intelligence in Investment
1.1 What is Artificial Intelligence?
Artificial intelligence refers to the simulation of human intelligence processes by machines, especially computer systems. This includes learning (the ability to improve performance based on past experiences), reasoning (drawing conclusions from available information), and self-correction.
1.2 The Role of AI in Investing
AI plays a pivotal role in the investment sector by:
- Analyzing market data and trends.
- Automating trading processes.
- Enhancing risk management.
- Personalizing investment strategies.
1.3 Types of AI Technologies Used in Investing
- Machine Learning: Algorithms that enable computers to learn from data and make predictions.
- Natural Language Processing (NLP): Allows machines to understand and interpret human language, often used for sentiment analysis in news and social media.
- Robotic Process Automation (RPA): Automates repetitive tasks such as data entry and report generation.
2. Setting Your Investment Goals
2.1 Define Your Objectives
Before employing AI tools, it’s crucial to clarify your investment goals. Common objectives include:
- Capital appreciation
- Income generation
- Risk management
- Diversification
2.2 Assess Your Risk Tolerance
Understanding your risk tolerance is vital in determining the appropriate AI strategies to employ. Consider your financial situation, investment horizon, and comfort with market volatility.
3. Gathering and Analyzing Data
3.1 Importance of Data in AI Investment
AI systems thrive on data. The quality and quantity of data can significantly influence the effectiveness of AI algorithms. Key data types include:
- Market Data: Historical prices, trading volumes, and market indices.
- Financial Statements: Company earnings reports, balance sheets, and cash flow statements.
- Alternative Data: Social media sentiment, news articles, and consumer behavior data.
3.2 Data Sources
- Financial Market Data Providers: Bloomberg, Reuters, and Yahoo Finance.
- Company Filings: EDGAR database for SEC filings.
- Alternative Data Platforms: Thinknum, AlphaSense, and similar services that provide insights from non-traditional data sources.
3.3 Data Cleaning and Preparation
Ensure that your data is clean and well-organized. This involves:
- Removing duplicates and irrelevant information.
- Filling in missing values or removing incomplete data.
- Normalizing data for consistency.
4. Leveraging Machine Learning for Investment Strategies
4.1 Developing Machine Learning Models
To leverage machine learning, you can develop models to analyze data and predict outcomes. Key steps include:
- Choose a Model: Common models include linear regression, decision trees, and neural networks.
- Training the Model: Use historical data to train your model, allowing it to learn patterns.
- Testing the Model: Validate the model’s predictions against a separate dataset to assess its accuracy.
4.2 Feature Engineering
Feature engineering involves selecting and transforming variables to improve model performance. Consider:
- Technical Indicators: Moving averages, relative strength index (RSI), and MACD.
- Fundamental Factors: Earnings growth, debt-to-equity ratio, and price-to-earnings (P/E) ratio.
- Sentiment Indicators: Social media sentiment scores and news article sentiment analysis.
4.3 Backtesting Your Strategy
Backtesting allows you to simulate your investment strategy using historical data. This process helps you understand how the strategy would have performed in the past and refine your approach.
5. Natural Language Processing in Investment
5.1 Sentiment Analysis
NLP can be used to gauge market sentiment by analyzing news articles, earnings calls, and social media posts. Tools like Python’s NLTK or libraries like TextBlob can help process and analyze textual data.
5.2 Event-Driven Investing
AI can identify events that may impact stock prices, such as earnings releases or major news announcements. By analyzing patterns related to these events, you can develop strategies to capitalize on market movements.
5.3 Automating Research
AI-powered tools can automate the research process, gathering insights from multiple sources, including analyst reports and social media trends, to inform your investment decisions.
6. Automated Trading and Robo-Advisors
6.1 Understanding Automated Trading
Automated trading systems use algorithms to execute trades based on predefined criteria. This approach can minimize emotional decision-making and ensure discipline in executing trades.
6.2 Implementing Algorithmic Trading Strategies
- Arbitrage: Exploiting price differences across markets.
- Market Making: Providing liquidity by placing buy and sell orders simultaneously.
- Trend Following: Identifying and trading in the direction of market trends.
6.3 Robo-Advisors
Robo-advisors are AI-driven platforms that provide automated investment advice. They typically assess your risk tolerance and investment goals to create and manage a diversified portfolio.
7. Risk Management with AI
7.1 Identifying Risks
AI can help identify various risks, including:
- Market Risk: Potential losses due to market fluctuations.
- Credit Risk: Risk of default by borrowers.
- Operational Risk: Risks arising from internal processes and systems.
7.2 Stress Testing
Stress testing involves simulating adverse market conditions to assess how your portfolio would perform under stress. AI can automate this process and provide insights into potential vulnerabilities.
7.3 Portfolio Optimization
AI can optimize your portfolio by analyzing historical performance, correlations between assets, and your risk tolerance to recommend the ideal asset allocation.
8. Ethical Considerations in AI Investment
8.1 Data Privacy
Ensure that the data used for AI analysis complies with regulations like GDPR and CCPA, safeguarding sensitive information.
8.2 Transparency and Explainability
Investors should strive for transparency in AI algorithms, understanding how decisions are made. Explainable AI can help demystify complex models and build trust in automated systems.
8.3 Avoiding Bias
AI models can inadvertently reflect biases present in the training data. Regularly audit your models to identify and mitigate potential biases.
9. Continuous Learning and Adaptation
9.1 Staying Updated on AI Trends
The field of AI is rapidly evolving. Stay informed about the latest advancements and trends through:
- Industry publications and blogs.
- Online courses and webinars.
- Conferences and networking events.
9.2 Iterative Improvement
Regularly assess and refine your AI models based on performance and changing market conditions. Continuous learning is key to maintaining a competitive edge.
9.3 Community Engagement
Engage with communities focused on AI and investing. Platforms like GitHub, Kaggle, and online forums can provide valuable insights, resources, and collaboration opportunities.
Artificial intelligence (AI), once the stuff of science fiction, is now making million-dollar investing decisions in milliseconds. In 2024, individual investors are left wondering: Is AI the key to unlocking unprecedented returns, or is it a double-edged sword that could reshape the financial landscape in unpredictable ways?
Integrating AI into investment portfolios is no longer reserved for tech giants and hedge funds. However, from stock selection algorithms to machine learning models that predict market trends, AI tools have become far more available to retail investors. These technologies can process vast amounts of data, help allocate portfolios, manage risk, and even provide personalized investment advice. Yet, as these AI applications proliferate, so do the questions surrounding how well they work and their long-term impact on the market. Are we on the cusp of a new era of data-driven investing, or are we inadvertently ceding control of our financial futures to algorithms we don’t fully understand?
Below, we explore the practical applications of AI in personal investment strategies for 2024. We’ll review how everyday investors are using these tools to try to improve returns and mitigate risks.
Stock Picking With AI
Investors have an overwhelming amount of data on all stocks traded on U.S. markets, which they examine to decide whether specific shares are worth buying or selling. AI potentially allows you to sort through this data to identify stocks that meet their criteria.
For example, stock screeners are sophisticated tools that filter stocks based on criteria that include fundamental and technical data points, such as accounting ratios, market capitalization, trading volume, and moving averages, to name just a few of the hundreds of data points available.
If you’re looking to get started with a stock screener, consider learning how to use these platforms by starting with one of the many free versions that are available, like ZACKS (Nasdaq).
AI-Automated Portfolios
Tan, Gordon Kuo Siong. “Robo-Advisors and the Financialization of Lay Investors.”Geoforum, vol. 117 (2020), pp. 46-60.
Robo-advisors like Wealthfront and Betterment automate the traditional process of working with an advisor to outline investing goals, time horizons, and risk tolerances to create a portfolio. Automated portfolios guide you through a questionnaire that then scores to a model portfolio that meets the criteria of the investor.
In addition to the questionnaire and the scoring of models, these platforms also use AI to determine the best mix of individual stocks for your portfolio. Automated portfolios can also be set to rebalance automatically should the target allocations in the portfolio drift too far from your original selections.
Managing Trades With AI
High-speed computing and near-instantaneous market trading has vastly changed how investors manage their trades in recent decades. Brokerage companies now offer customers sophisticated AI-powered order entry tools that can monitor and execute trades based on your criteria. This automated approach to trade management can significantly improve your trades.
For example, an AI-driven system can be programmed to do the following:
- Buy a stock when it meets the conditions you set.
- Manage exit strategies using conditional orders.
- Carry out stop-loss orders or take-profit levels based on real-time market conditions.
A major advantage of using AI for trade management is its potential to mitigate the emotional aspects of trading. By adhering to preset rules and criteria, AI systems can help you keep disciplined and avoid impulsive decisions that can ruin your long-term strategies. This emotional detachment can be particularly valuable in volatile market conditions, where human emotions often lead to rash trading.
However, it’s crucial to remember that while AI can help manage your trades, it should be used judiciously. You still have to understand the underlying strategies and regularly review and adjust your AI trading criteria to ensure they align with your investment goals and risk tolerance
Portfolio Optimization
AI is a good tool for improving a portfolio, allowing you to identify a portfolio that fits your specific needs, including your risk tolerance and time horizon. In addition, once you select a particular type of portfolio, a platform’s AI can be used with modern portfolio theory to choose stocks and other assets that fall on the efficient frontier. This is a set of optimal portfolios that offer the highest expected return for a preset level of risk.
Managing Risk With AI
AI can optimize portfolios to lie on an efficient frontier, hopefully putting expected returns at the level of risk you’re willing to accept. These systems can also monitor and alert you when you’re overexposed to individual stocks or sectors.
Here’s a look at how AI can be used to bolster classic ways of managing risk:
Managing risks for specific trades: AI-powered tools can carry out complex order strategies, such as conditional orders, stop-losses, and take-profit levels, to manage risk on active trades. In addition, AI programs can design and automate options strategies for hedging and income generation, adjusting these strategies in real time based on market conditions.
Sophisticated risk analysis: AI can run millions of simulations to assess portfolio risk under various market scenarios, providing a more comprehensive view of potential outcomes. It can also subject portfolios to stress tests, helping you understand how your investments might perform during market crises.
Dynamic risk adjustment: AI systems can continuously analyze market data, news, and alternative data sources to detect risks early. Machine learning algorithms can adapt risk models in real time, accounting for changing market dynamics and correlations.
Behavioral risk management: Algorithmic trading powered by AI can help reduce the emotional aspect of trading. AI systems can implement preset rules, helping you stick to your risk management strategies even in volatile markets.
Regulatory compliance: AI can ensure that portfolios remain compliant with laws and regulations, as well as your mandates, automatically flagging or adjusting for potential violations.
Tail risk management: AI models can attempt to identify potential extreme events that traditional models might miss, helping prepare for tail risks, also known as “black swan” events.
How Robo-Advisors Use Artificial Intelligence
Through automated portfolio building, robo-advisors automate the traditional process of working with an advisor to outline investing goals, time horizons, and risk tolerances to create a portfolio that meets the needs of the investor.
Automated portfolios guide the user through a questionnaire that then scores a model portfolio that meets the investor’s criteria. In addition to the questionnaire and the scoring of models, these platforms also use AI to determine the best mix of individual stocks for the portfolio, which is often accomplished using modern portfolio theory. Further, automated portfolios are also set to automatically rebalance if the target allocations drift too far from the selected portfolio.
Steps for Using Artificial Intelligence While Investing
If you want to incorporate AI into your investing or trading, you may consider taking the steps that follow.
Step 1: Set Out Your Financial Goals
The first step is the same for every investor, which is to understand your financial goals so you can move forward with an investment strategy that fits your needs.
Step 2: Choose Your Investing Method
Next, you need to determine whether you’ll use a robo-advisor that does much of the work or invest on your own. If you go with a robo-advisor, the advisor’s AI technology will do the heavy lifting. You’ll answer questionnaires, review model proposals, and give further input on portfolio management.
Step 3: Select an Investing Strategy
If you’re deciding on the investments, you’ll need to determine your strategy to understand the types of stocks you want. You can also use suggested models from robo-advisors, often available for free, to help determine the mix of asset classes for their portfolio. This uses AI to help set a strategy you’ll manage individually.
Step 4: Identify Your Investing Tools
Stock screeners are helpful AI tools when choosing individual stocks for your portfolio. These often have preset filters to help you get started.
Step 5: Start Managing Your Portfolio
Once the portfolio is up and running, you can employ different automated tools to help manage your positions to enter and exit your positions. You might also want to refine your stock screen searches and learn to use the efficient frontier to craft a portfolio for favorable returns and the lowest risk possible.
10. Conclusion
Leveraging artificial intelligence to enhance your investment strategies can provide a significant advantage in today’s complex financial landscape. By understanding how to gather and analyze data, develop machine learning models, and implement automated trading strategies, you can invest like an expert. As AI continues to evolve, staying informed and adaptable will be crucial to your success in the investment world.